How Should You Collect Maintenance Data That Matters Most?
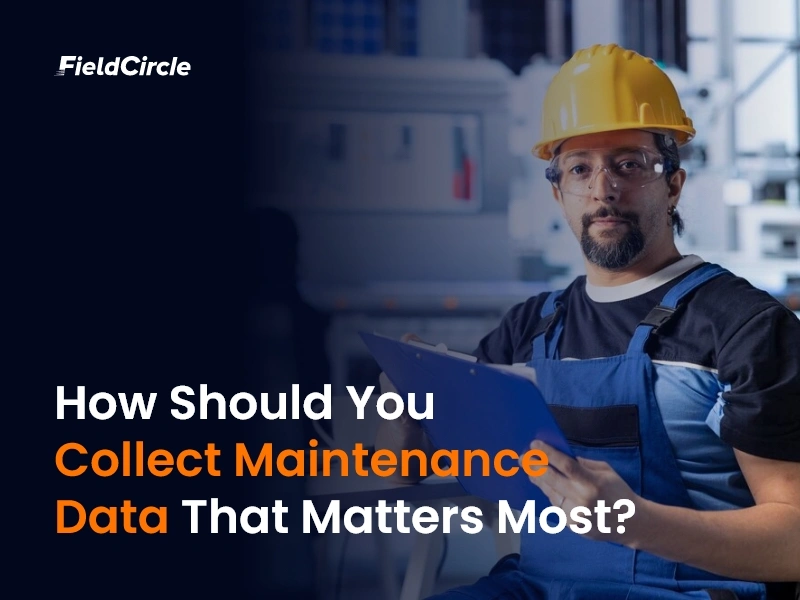
On average unplanned downtime costs industries $260,000 per hour—a figure that could cripple profitability, erode stakeholder trust, and even shutter unprepared organizations.
For maintenance leaders, managers, and top executives, having the right maintenance data at their fingertips isn’t just helpful—it’s essential for driving efficiency and cutting costs. Yet, many organizations struggle with gathering data that truly informs decision-making.
Maintenance data encompasses the vital information that describes how your equipment is performing—from sensor readings and work order histories to metrics like Mean Time Between Failures (MTBF) and Mean Time to Repair (MTTR). When captured correctly, this data empowers you to shift from reactive “fix it when it breaks” approaches to proactive, predictive maintenance strategies.
Industry analysts have long emphasized the advantages of utilizing quality maintenance data. For instance, McKinsey reports that companies adopting predictive maintenance strategies can reduce unplanned downtime by up to 50% and cut maintenance costs by as much as 40%.
The key maintenance metrics that drive actionable insights include:
- MTBF: A higher MTBF indicates more reliable equipment, helping you plan maintenance schedules effectively.
- MTTR: A shorter MTTR means your team can quickly resolve issues, minimizing production losses.
- Overall Equipment Effectiveness (OEE): This composite metric captures availability, performance, and quality to give a holistic view of asset management.
- Preventive Maintenance Compliance (PMC): Tracking the percentage of scheduled tasks completed on time ensures that you’re staying ahead of potential failures.
Understanding these metrics and how they correlate with your operational goals is the first step toward building a maintenance strategy that transforms raw data into business value.
Common Challenges in Collecting Maintenance Data
Despite its importance, many organizations face significant hurdles when it comes to collecting maintenance data that’s truly useful. Here are some of the most common challenges:
- Data Overload and Irrelevance: Maintenance teams are often bombarded with a mountain of data from various sensors and systems. Yet, not every piece of information is actionable. The sheer volume can make it difficult to sift through noise and identify what’s truly indicative of equipment health.
- Fragmented Systems and Inconsistent Practices: In many organizations, maintenance data is trapped in silos. Different systems—be it computerized maintenance management system (CMMS) platforms, spreadsheets, or standalone sensor networks—often don’t “talk” to one another. This fragmentation results in inconsistencies and gaps, making it nearly impossible to build a comprehensive picture of your assets’ conditions.
- Lack of Standardization and Training: Even when data is collected, it’s not always done uniformly. Inconsistent methodologies, varying units of measure, and ad hoc data entry practices can lead to unreliable or even misleading insights. Many teams also lack the proper training to capture and interpret data accurately, which further undermines its value.
- The Impact on Operations: When data collection falls short, the consequences are far-reaching. Poor data leads to reactive maintenance strategies that drive up costs, extend downtime, and even compromise safety. Without accurate, reliable data, maintenance leaders struggle to justify budgets or pinpoint areas in need of improvement, leaving organizations to operate in a reactive mode that can be both costly and frustrating. On the other hand, with the right data they can strategically approach maintenance budgeting and other key activities.
The Ripple Effects of Poor Data Collection
Poor data collection doesn’t just lead to unplanned downtime—it creates a cascade of challenges:
- Reactive Maintenance Culture: When you can’t trust your data, you’re forced to adopt a “fix it after it breaks” mentality, which is inherently inefficient and expensive.
- Increased Costs: Unplanned repairs are not only disruptive but often far more costly than scheduled maintenance. Over time, these reactive measures can erode profit margins.
- Safety and Compliance Risks: Inaccurate data can mask warning signs of equipment degradation, putting both employees and the environment at risk.
- Strategic Missteps: For top management, the inability to present clear, data-driven insights hampers informed decision-making. This can affect everything from capital investments in new equipment to workforce planning and operational improvements.
For maintenance leaders, these challenges are all too real. The frustration of pouring over data that doesn’t lead to actionable insights is a constant headache—and one that can ultimately compromise the organization’s competitive edge.
Best Practices for Collecting Relevant Maintenance Data
So, how do you overcome these challenges? The solution lies in adopting a structured approach to data collection that ensures every piece of information you capture is both accurate and actionable. Here are some strategies to consider:
1. Define Clear Objectives Aligned with KPIs
Before you start collecting data, ask yourself, “What decisions will this data support?” Identify the key performance indicators (KPIs) that matter most—whether it’s reducing downtime, extending asset life, or lowering maintenance costs. Align your data collection efforts with these objectives. For example, if your primary objective is to reduce costly unplanned downtime, your focus should be on capturing data that directly influences essential maintenance metrics such as Mean Time to Repair (MTTR) and Mean Time Between Failures (MTBF).
Action Steps:
- Identify Key Performance Indicators (KPIs): Work with your maintenance, operations, and finance teams to pinpoint the KPIs that matter most.
- Establish Data-Driven Goals: Set specific, measurable targets. For example, “Reduce unplanned downtime by 30% over the next 12 months” or “Improve MTBF by 15% within the next quarter.”
- Map Data Requirements to Objectives: Once your KPIs and targets are clear, determine what type of data is necessary. This might include sensor readings, historical work order data, or operator reports. The goal is to ensure that every piece of data collected has a direct link to an operational or strategic objective.
By defining clear objectives and aligning them with measurable KPIs, you lay the groundwork for a focused data collection effort that eliminates unnecessary information and emphasizes what truly drives performance improvement.
2. Standardize Data Collection Processes
Implement uniform data collection methods across your organization. Standardized forms, protocols, and sensor calibration procedures ensure that data is consistent and comparable over time. Establish clear guidelines on what data needs to be captured, in which format, and at what frequency. This standardization not only improves accuracy but also simplifies the integration of data from multiple sources.
Detailed Strategies Include:
- Develop Uniform Protocols: Create and document standard operating procedures for data collection. This should detail how to record data (e.g., unit measurements, frequency of collection, data formats), ensuring consistency across shifts and departments.
- Implement Data Templates and Checklists: Utilize standardized forms and digital templates for logging maintenance activities, sensor readings, and work orders. For example, every work order could include standardized fields such as equipment ID, time of failure, duration of downtime, and corrective action taken.
- Use Automated Tools Where Possible: Integrate automation in data collection to reduce human error. For instance, use barcode scanners or mobile applications that enforce predefined data entry rules, ensuring that every data entry adheres to the established format.
- Regular Audits and Quality Checks: Schedule periodic reviews of the collected data to identify discrepancies or deviations from the standards. Implement validation rules (such as range checks or consistency checks) in your systems to automatically flag data that falls outside acceptable parameters.
By standardizing the data collection process, you ensure high data quality and facilitate seamless integration across different systems and teams. This uniformity is critical when it comes time to analyze data and derive actionable insights.
3. Leverage IoT and Automation
With the help of modern CMMS, sensors and IoT devices can collect data in real time, reducing the burden of manual entry and minimizing human error. The ecosystem brings up-to-date data in one centralized location and this is how automation allows for continuous monitoring. The real-time data is invaluable for predictive maintenance—enabling you to identify early warning signs of failure and take corrective action before a breakdown occurs.
Key Considerations:
- Real-Time Data Acquisition: Deploy IoT sensors that continuously monitor parameters such as temperature, vibration, pressure, and humidity. For example, sensors on critical machinery can automatically transmit data to a centralized system in real time, allowing for immediate detection of anomalies.
- Integration with Maintenance Systems: Connect these IoT devices with your CMMS system. This integration allows for the seamless flow of data, ensuring that insights are immediately actionable.
- Reduce Human Intervention: Automated data collection minimizes the need for manual input, which not only saves time but also reduces the risk of human error like misspelled part names, inconsistent abbreviations, or missing timestamps. With automation, maintenance teams can focus more on analysis and decision-making rather than data entry.
- Scalability: As your operations grow, IoT solutions can be scaled up quickly to cover new assets or processes without significantly increasing the workload for your staff.
Using IoT and automation transforms maintenance data collection from a periodic, manual task into a continuous, and reliable maintenance process.
4. Integrate Systems to Break Down Silos
Don’t let valuable data remain trapped in isolated systems. Integrate your CMMS with other operational systems to create a centralized data repository. This unified approach allows you to see the full picture of your asset performance, making it easier to derive actionable insights and streamline decision-making.
To Achieve Seamless Integration:
- Centralized Data Repositories: Implement a centralized database or data lake where all maintenance data—whether from sensors, manual logs, or historical records—can be stored and accessed uniformly.
- API and Middleware Solutions: Use APIs and middleware to connect disparate systems. For example, integrate your CMMS with ERP, IoT platforms, and even legacy systems to ensure that data flows freely between them.
- Data Mapping and Normalization: Before integrating, map the data from each system to a common schema. This process involves normalizing different data formats and units so that comparisons and correlations can be made accurately.
- Real-Time Data Synchronization: Aim for real-time or near-real-time data synchronization across systems to avoid delays and ensure that the latest information is always available for decision-making.
By breaking down silos, your organization can consolidate data from various sources, making it easier to analyze trends, identify issues, and take proactive maintenance actions based on a complete picture of your operations.
5. Focus on Key Metrics
Instead of trying to capture every possible data point, hone in on the metrics that truly matter. Prioritize indicators like MTBF, MTTR, OEE, and PMC. By concentrating on these core metrics, you can more effectively gauge the health of your equipment and the efficiency of your maintenance processes.
Implementation Strategies:
- Prioritize Core Metrics: Concentrate on high-impact metrics such as MTBF, MTTR, Overall Equipment Effectiveness (OEE), and Preventive Maintenance Compliance. These metrics provide a clear picture of equipment reliability, efficiency, and the effectiveness of maintenance schedules.
- Align Metrics with Business Objectives: Ensure that each metric is tied directly to a business outcome. For example, if reducing downtime is a priority, then metrics like MTTR should be monitored closely.
- Develop Custom Dashboards: Use advanced analytics tools to create dashboards that track these core metrics in real time. Dashboards should be customizable so that different stakeholders can view the metrics most relevant to their roles.
- Benchmarking: Compare your performance against industry standards or historical data to determine where improvements are needed. Benchmarking provides a context for the data and helps in setting realistic, achievable targets.
By focusing on key metrics, you avoid data overload and ensure that the insights derived are directly aligned with your strategic maintenance goals.
6. Train Your Team
Even the best technology is only as good as the people who use it. Ensure that your maintenance team is well-trained in data collection and analysis techniques. Regular training sessions and clear documentation can empower your staff to collect data accurately and interpret it effectively, leading to better overall performance.
Training Strategies:
- Comprehensive Onboarding Programs: When new technologies or data collection protocols are introduced, conduct detailed training sessions that explain both the technical aspects and the strategic importance of accurate data collection.
- Regular Refresher Courses: The technology landscape and operational processes evolve rapidly. Regular refresher courses and workshops can keep your team updated on best practices, new tools, and emerging analytical methods.
- Hands-On Training and Simulations: Use simulations and practical exercises to help staff understand how data errors can affect decision-making. For example, demonstrate how misreported sensor data might lead to incorrect maintenance schedules or misallocation of resources.
- Cross-Departmental Collaboration: Cross-train your maintenance team and encourage collaboration between the maintenance department and other important functions such as IT, and data analysts to foster a culture of data literacy across the organization. This collaboration helps ensure that insights drawn from data are understood and implemented effectively.
Empowering your team through training not only improves the quality of data collected but also enhances the overall responsiveness and effectiveness of your maintenance operations.
7. Use Advanced Analytics for Actionable Insights
Collecting data is only half the battle—the real value comes from turning that data into actionable insights. Utilize data analytics tools to process and visualize your maintenance data. Dashboards and automated reporting can highlight trends, pinpoint inefficiencies, and even forecast potential failures. This proactive approach enables you to plan maintenance activities more effectively, reducing unplanned downtime and optimizing resource allocation.
To Harness Advanced Analytics:
- Develop Predictive Models: Use historical maintenance data to build predictive models that can forecast equipment failures or identify conditions that precede downtime. For example, machine learning algorithms can be trained to recognize patterns that indicate wear and tear or impending failure.
- Visualize Data Effectively: Create interactive dashboards that not only display real-time metrics but also offer drill-down capabilities. This allows maintenance managers to explore the underlying data, understand trends, and make informed decisions.
- Set Up Automated Alerts: Configure your analytics systems to trigger alerts when metrics deviate from acceptable thresholds. For instance, if sensor data indicates that a machine’s vibration levels are abnormal, an automated alert can prompt immediate investigation.
- Integrate Business Context: Ensure that the analytical models incorporate business context by linking technical data with operational outcomes. This might involve correlating sensor data with production output, energy usage, or cost savings, thereby providing a comprehensive view of how maintenance activities impact overall business performance.
- Continuous Improvement: Analytics should not be a one-time exercise. Establish a continuous improvement loop where the models are regularly updated with new data, and the insights are used to refine maintenance schedules, resource allocation, and strategic planning.
By leveraging advanced analytics, organizations can move beyond descriptive reporting to predictive and prescriptive insights, enabling proactive maintenance strategies that prevent failures, optimize resource utilization, and drive cost savings.
Turn Data Into Action
Take the first step towards transforming your maintenance strategy by collecting, analyzing, and acting on the data that matters most. A maintenance management software is a great lever in this process. Once you have established processes to capture high-quality data, the next step is to ensure that this information drives real-world decisions.
Regularly review your data collection processes and analytical models to ensure they align with your organizational goals. Stay open to refining your approach based on new technologies, changing needs, or team feedback. Invest in the right tools and nurture a data-driven culture that transforms maintenance data into a powerful tool for operational excellence.