Top Technologies Used in Condition-Based Maintenance
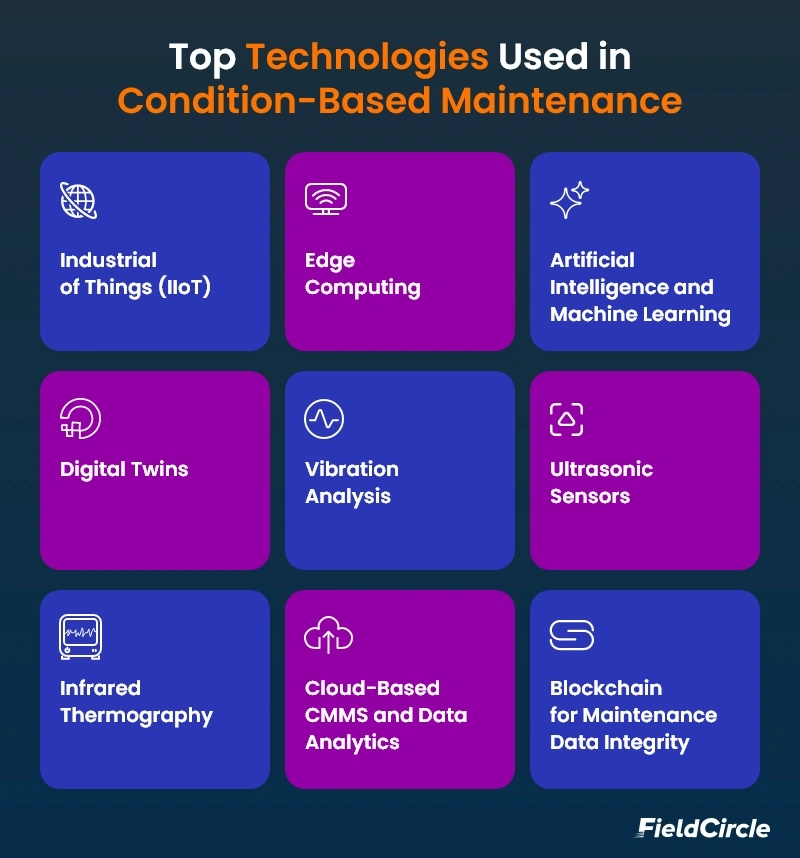
Condition-based maintenance is an important maintenance technique which relies heavily on the use of various technologies.
The need for technology stems from the fact that monitoring equipment based on its actual condition requires assessing multiple parameters, from temperature and vibration to pressure and fluid levels. And technology simplifies this process by collecting real-time data, identifying patterns, and detecting anomalies.
Notably, technological innovations have progressed from basic sensors to AI-driven analytics and Industrial IoT, making condition monitoring systems more precise and reliable.
Every maintenance professional must be aware of the useful technologies to build a smart maintenance function. So we discuss noteworthy technologies that are revolutionizing condition-based maintenance (CBM).
-
Industrial of Things (IIoT)
At the heart of CBM is the ability to collect real-time data from machines, and this is made possible by IIoT devices. IoT sensors (temperature probes, vibration accelerometers, pressure transducers, etc.) are installed on critical equipment to continuously monitor conditions. These smart sensors stream a wealth of data about how assets are performing right now.
The real-time visibility offered by IIoT is a game-changer as it helps maintenance teams catch anomalies easily. Technicians get a heads-up to intervene and they don’t have to wait for failure. For instance, IoT vibration sensors on a rotating machine can establish a “normal” vibration signature. If the pattern deviates – which signals imbalance or a bearing starting to fail – the system alerts the team immediately.
In this way, IoT-enabled sensors bring a new level of precision and speed to maintenance: they deliver continuous telemetry on equipment health, enabling an immediate and informed response to any irregularities.
-
Edge Computing
While you need IIoT to create a connected ecosystem, you also need an apt mechanism to utilize the data generated by IIOT. This is where edge computing comes into play. Edge computing processes data closer to where it’s generated (e.g. on a device or local gateway at the factory), rather than sending every raw data point to a cloud or central server.
If we consider a manufacturing business with several manufacturing facilities with thousands of sensors, raw data streams can be overwhelming in volume and too time-sensitive to rely on distant cloud analysis. By filtering and analyzing data at the edge, companies can react quickly to critical events and reduce network load.
In a nutshell, the IIoT + Edge combo provides the nervous system for modern CBM sensing equipment condition in real time and acting on insights quickly on-site, with essential data forwarded to cloud analytics for deeper analysis.
-
Artificial Intelligence and Machine Learning
Collecting data is only one part of the CBM, making sense of that data is the other critical piece. This is where Artificial Intelligence (AI) and Machine Learning (ML) step in. AI algorithms can sift through volumes of sensor readings and learn patterns that correspond to normal vs. faulty operation.
Over time, a machine learning model “trains” on historical data of both healthy and failed states, enabling it to recognize the subtle signatures of an impending failure that humans might miss. AI-driven failure prediction can far outperform simple thresholds. These algorithms continuously improve as they get more data, adapting to each asset’s behavior.
The power of AI and ML in maintenance is that it can handle the complexity and volume of maintenance data that would overwhelm maintenance professionals and identify non-obvious correlations and subtle trends.
In practice, AI models are giving rise to predictive maintenance. They are used to predict remaining useful life (RUL) of components, and perform advanced anomaly detection on complex equipment (like detecting an early-stage pump cavitation or an incipient bearing failure from vibration frequency patterns). And the use cases about.
-
Digital Twins
A digital twin is a virtual replica of a physical asset that mirrors the equipment’s state in real time. In the context of condition-based maintenance, digital twins allow maintenance teams to visualize and experiment with an asset’s condition in ways that aren’t possible with the physical machine running on the plant floor.
The twin receives input from all the machine’s IoT sensors and operational data, so it reflects temperature, pressure, speed, vibration, and other metrics just like the real asset at that moment. With all necessary information at hand, you get a holistic, real-time view of equipment health.
Beyond monitoring, one of the biggest advantages of digital twins in CBM is its ability to simulate scenarios without risking the actual equipment. Maintenance engineers can test “what-if” scenarios. For instance, they can check how the machine would behave if a certain part degrades further or if load is increased, and see potential failure modes in the virtual model.
Digital twins can also leverage historical data and can compare current readings to prior baseline conditions or even run predictive models to forecast future behavior. In essence, the twin not only mirrors the present, but can project into the future.
-
Vibration Analysis
Not all technologies in condition-based maintenance (CBM) are brand new, and techniques like vibration analysis have been stalwarts of condition-based monitoring for decades, and they remain absolutely critical in the modern CBM toolbox. It measures the vibration levels and frequencies of machinery to assess its health using accelerometers and velocity transducers.
Mechanical issues such as imbalance, misalignment, bearing wear, mechanical looseness, resonance, or bent shafts will all alter a machine’s vibration signature in characteristic ways. By capturing vibration data and analyzing the frequency spectrum, maintenance specialists can detect these faults very early – often weeks or months before equipment failure.
Modern CBM systems can continuously monitor vibration and with AI capabilities can automatically diagnose issues. This is a shift from doing monthly manual vibration readings to having a sensor permanently on the asset and software that flags any concerning changes immediately.
-
Ultrasonic Sensors
Ultrasonic analysis, using acoustic or ultrasonic sensors, is an effective condition-based maintenance (CBM) technology. It detects high-frequency sounds beyond human hearing, allowing for the identification of issues caused by friction, turbulence, or electrical discharges.
Ultrasonic detectors are particularly useful for locating pressure or vacuum leaks in compressed air and gas systems, as small leaks emit an ultrasonic hiss. This technology helps maintenance personnel identify leaks that could otherwise lead to energy loss.
In electrical systems, ultrasonic listening can detect arcing or corona discharges before they become visible problems, complementing infrared thermography. Additionally, it can monitor steam traps – a malfunctioning steam trap emits a distinct rattling sound indicative of steam leakage.
These technologies, while mature, are actively being enhanced with IoT connectivity and AI analytics. They form an indispensable bridge between the physical behavior of machines and the digital analysis that triggers preventive actions.
-
Infrared Thermography
Another crucial technology in the CBM is infrared thermography. Here, thermal imaging cameras (or IR sensors) detect heat patterns on equipment. Excess heat is a red flag, and denotes friction due to lack of lubrication, an overloaded electrical circuit, insulation failure, or other developing problems.
Infrared thermography allows maintenance teams to literally see the heat – it converts emitted IR radiation into a visual temperature map (thermogram) of the asset. By comparing current thermograms to baseline images, you can spot abnormal hot spots or temperature differentials very clearly.
Thermography is widely used for monitoring motors, gearboxes, and bearings, checking for excessive heat that signals friction or impending failure. It’s also invaluable for inspecting refractory linings and insulation as well as checking fluid levels in sealed vessels (different fill levels show different heat distribution).
One major advantage of infrared inspections is that they are non-contact and can be performed while equipment is running (no shutdown needed). A technician can walk around with a thermal camera or set up a fixed thermal sensor and immediately identify components operating above their normal temperature range. IR scans are also fast, you can survey a large area or many components in a single view, making it efficient to regularly audit the “thermal health” of a plant.
-
Cloud-Based CMMS and Data Analytics
As maintenance data volume explodes from all these sensors and devices, industrial organizations are increasingly leveraging cloud-based platforms to manage and analyze information.
Modern cloud-based Computerized Maintenance Management Systems (CMMS) store and process maintenance schedules, work orders, asset histories, and now even real-time condition data on remote servers accessible via the web. Their use brings down the downtime of equipment which continues to work ceaselessly.
Cloud infrastructure offers two big advantages for CBM: scalability and centralized analytics. A cloud platform can handle enormous data volumes, scaling up storage and computing resources.
Centralized analytics and accessibility is the other benefit. With data and systems in the cloud, maintenance managers and reliability engineers can access dashboards from anywhere. Powerful data analytics tools in the cloud can correlate data from different sources – production data, maintenance logs, and condition sensors to find deeper insights.
Cloud platforms also ease the integration challenge: many legacy systems can be bridged to cloud services via gateways, and cloud APIs allow different applications to share data. This means your CBM solution, ERP, and spare parts inventory system could all tie together seamlessly in the cloud.
-
Blockchain for Maintenance Data Integrity
A more nascent but intriguing technology in maintenance is blockchain, primarily used to ensure data integrity and trust in maintenance records. Blockchain is essentially a decentralized, tamper-proof ledger. Once data is recorded on a blockchain, it cannot be altered without leaving a trace, thanks to cryptographic linking of blocks.
In maintenance, blockchain can be applied to equipment service records, sensor data logs, and even warranty or compliance documents. From a CBM perspective, blockchain could secure sensor data streams as well – ensuring that the data feeding your AI models is authentic (not spoofed or tampered). This is important as maintenance decisions become more automated, you want confidence that the trigger (data) is valid.
Another use case is smart contracts for maintenance. For instance, a service contract between a company and an OEM could be encoded on a blockchain: when sensor data indicates that a certain threshold is passed (say a part has worn to a certain level), it can automatically trigger a service request or payment as per the contract terms.
To Wrap Up
You must deploy technologies if you want to optimize maintenance costs by reengineering the processes. No two ways about it.
While adopting and implementing them requires new skills and initial investment, as we saw, it results in more efficient and proactive maintenance.
The real advantage of CBM technologies is that they allow teams to focus on preventing issues rather than just reacting to them, where preventive maintenance software proves to be a handy tool. Start embracing these technologies at the earliest for a state-of-the-art maintenance function.